Activity Recognition and Event Detection in Table Tennis
Activity Recognition and Event Detection in Table Tennis
(Own Funds)
Project leader: ,
Project members:
Start date: 1. January 2015
URL: https://www.mad.tf.fau.de/research/projects/esifitness/activity-recognition-and-event-detection-in-table-tennis/
Publications:
Description
Recent advances in microelectronics, sensor integration and data analysis techniques opened new possibilities for wearable technology (Wearables) and found its way into health, fitness and sports applications and device to be integrated into different body-worn accessories such as garments, wristbands, shoes, smartphones or sports equipment like balls or rackets. Here, we specifically investigated table tennis, because its many possible strokes to achieve spin and speed variations, definitely make it an interesting and promising candidate for research. Furthermore, many variations are possible due to a huge amount of rubbers and rackets. Moreover, different grip styles such as shakehand or pen-holder have become accepted. Despite all these variations, sensor-based approaches can be used to develop a complete table tennis support system and match analysis tool.
Sensor-based Stroke Detection and Stroke Classification
We attached inertial sensors to table tennis rackets and collected data of 8 different basic stroke types from amateur and professional players. Firstly, single strokes were detected by a event detection algorithm. Secondly, features were computed and used as input for stroke type classification. Multiple classifiers were compared regarding classification rates and computational effort. Due to classification rates over 95% this approach is able to detect table tennis strokes in time-series data and to classify each stroke into correct stroke type categories. The system has the potential to be implemented as an embedded real-time application for other racket sports, to analyze training exercises and competitions, to present match statistics or to support the athletes’ training progress.
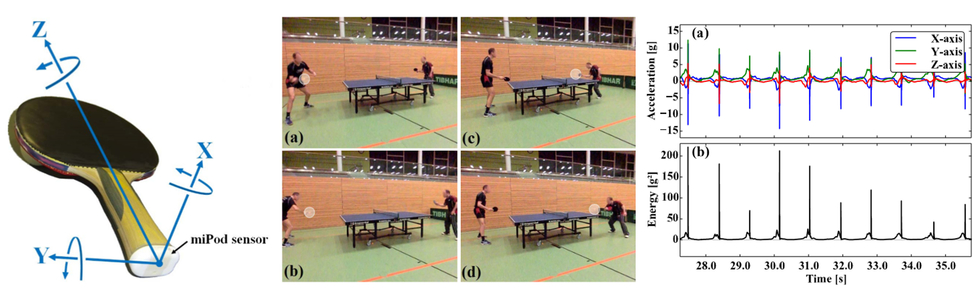
Ball Impact Localization using Piezo-electric Vibration Sensors
Three sensors were used as vibration sensors at the outer edge of the racket. We analyzed ball impact vibration appearances and measured time differences of arriving wave fronts for each sensor pair. A study was conducted comprising six different racket-rubber combinations. Firstly, we calculated a time difference distribution model based on training sets of impact data constructed by a grid of measuring points. Secondly, this model was used to estimate the impact position of test data sets using three different methods. Best performance yielded a RMSE of around 20 mm. The measuring system is small enough to be completely invisibly integrated into table tennis rackets and has the potential to be used in real-world training exercises and competitions to assist table tennis players during matches and to support the athletes’ training progress.
Ball Spin and Speed Estimation
We try to estimate the ball speed and spin estimation table tennis only based on a single racket-mounted inertial sensor. We conducted a research study comprising eight subjects performing different stroke types resulting in various ball speeds and spins. All ball-racket impacts were recorded with a high-speed camera for further evaluation. Firstly, several assumptions and simplifications of the unknown initial ball properties and the movements of player and racket had to be made. Secondly, the racket blade velocity right after impact was calculated. This was combined with a rebound model between the ball and the rubber to predict the speed and spin of the ball. Overall, the ball speed of strokes with forward spin could be estimated with an accuracy of 79.4 % and for strokes with backward spin with an accuracy of 87.4 %. The spin was estimated with an accuracy of 73.5 % and 75.0 %, respectively.
Further developments:
⇒ stroke detection using sound sensors
⇒ embedded racket implementation
Publications
Ball Speed and Spin Estimation in Table Tennis Using a Racket-mounted Inertial Sensor
ACM International Symposium on Wearable Computers (Maui, Hawaii, USA, 11. September 2017 - 15. September 2017)
In: Proceedings of the 2017 ACM International Symposium on Wearable Computers 2017
DOI: 10.1145/3123021.3123040
BibTeX: Download , , :
Sensor-based stroke detection and stroke type classification in table tennis
The 2015 ACM International Joint Conference on Pervasive and Ubiquitous Computing (Osaka, 7. September 2015 - 11. September 2015)
In: Proceedings of the 2015 ACM International Symposium on Wearable Computers 2015
DOI: 10.1145/2802083.2802087
BibTeX: Download , , , :
Ball impact localization on table tennis rackets using piezo-electric sensors
The 2016 ACM International Joint Conference on Pervasive and Ubiquitous Computing (Heidelberg, Deutschland, 12. September 2016 - 16. September 2016)
In: Proceedings of the 2016 ACM International Symposium on Wearable Computers 2016
DOI: 10.1145/2971763.2971778
BibTeX: Download , , :